|
Tutorial on Evaluation Indices of Statistical Performance: A Review Study |
|
Farzan Madadizadeh 1 , Hooman Yekrang Safakar 2 , Bita Forootani 2 ,
Malihe Bolukyazdi 2 , Zohreh Khosravani Shooli 2 , Sajjad Bahariniya 3*
- Departments of Biostatistics and Epidemiology, School of public health, Shahid Sadoughi University of Medical Sciences, Yazd, Iran
- Department of Nutritional Sciences, School of Public Health, Shahid Sadoughi University of Medical Sciences, Yazd, Iran
- Department of Health Services Management, School of Public Health, Shahid Sadoughi University of Medical Sciences, Yazd, Iran
ARTICLE INFO |
|
ABSTRACT |
Review article
Received: 20 September 2022
Accepted: 29 March 2023
|
|
Statistical indicators are essential parts of research in many scientific fields such as health and treatment. These indicators play a major role in the evaluation of many health indicators in the general population and can help predict future issues. Statistical indicators are needed to evaluate performance of the tests. Two of the primary indicators are sensitivity and specificity, and other indices are obtained from them. In this tutorial study, evaluation indicators of statistical performance such as false negative rate (FNR), false positive rate (FPR), false discovery rate (FDR), false omission rate (FOR), bookmaker informedness (BM), markedness (MK), diagnostic odds ratio (DOR), positive likelihood ratio (PLR), negative likelihood ratio (NLR), prevalence threshold (PT), threat score (TS), prevalence (P), Fowlkes-mallows (FM), Phi-coefficient or Matthews correlation coefficient (MCC) and F1 score have been reviewed.
Keywords: Data Accuracy, Likelihood Ratio Test, Sensitivity and Specificity, Statistics |
|
|
|
Corresponding Author:
Sajjad Bahariniya
sajjadbahari98@gmail.com
|
How to cite this paper:
Madadizadeh F, Yekrang Safakar H, Forootani B, Bolukyazdi M, Khosravani Shooli Z, Bahariniya S. Tutorial on Evaluation Indices of Statistical Performance: A Review Study. J Community Health Research 2023; 12(1): 25-29.
Introduction
Today, it is no secret that statistics play an important role in various fields of medicine and health. Statistical indicators (SI) are an example in this regard. SI such as sensitivity and specificity and their derivatives are used as performance indicators of many tests in medical science, so they can provide useful information to researchers (1- 3).
In this tutorial, first, the two main concepts of sensitivity and specificity are discussed, and then, the indicators derived from them will be explained in detail. Sensitivity and specificity, from a statistical point of view, express the performance of a test regarding the presence or absence of a disease (4). Sensitivity shows how well a test can identify true positive cases; specificity shows how well a test can identify true negative cases (5, 6).
If the true state of the condition cannot be identified, sensitivity and specificity can be defined relative to the "gold standard test". There is usually a relationship between sensitivity and specificity, such that higher sensitivity means lower specificity.(7). A test that leads to a high number of true positives and a low number of false negatives in diagnosing the condition has high sensitivity (8). A test that results in a high number of true negatives and a low number of false positives has a high specificity. This is important when people are diagnosed with the disease (3).
Imagine a study in which the results of a test are used to screen sick people. The test result can be positive or negative. The test results for each subject may or may not correspond to the actual conditions of the subject. Therefore, the following results might be obtained:
True positives: People who are correctly identified as sick. False positives: people who are wrongly identified as sick. True negative: People who are correctly identified as healthy. False negatives: people who are wrongly identified as healthy.
After obtaining the numbers of true positives, false positives, true negatives and false negatives, sensitivity and specificity can be calculated.
Table 1. Indicator’s guide
# Positive (P) |
The number of real positive cases |
# Negative (N) |
The number of real negative cases |
True positive (TP) |
A test result which correctly indicates the presence of a condition |
True Negative (TN) |
A test result which correctly indicates the absence of a condition |
False positive (FP) |
A test result which wrongly indicates a particular condition or attribute is present. |
False negative (FN) |
A test result which wrongly indicates a particular condition or attribute is absent. |
Sensitivity, recall, hit rate (HR), or true positive rate (TPR)
Sensitivity refers to the ability of a test to correctly distinguish patients from healthy individuals. Sensitivity is the result of dividing true positives by the sum of true positives and false negatives (9). Mathematically, this can be expressed as:
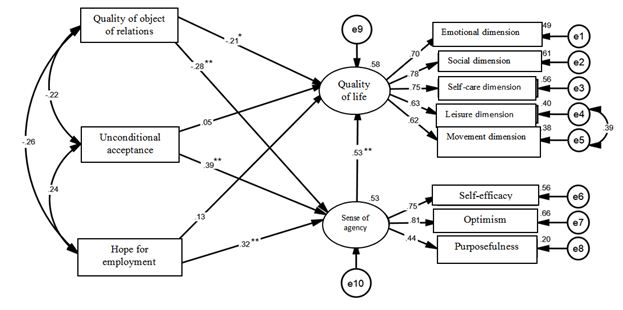
Specificity, selectivity or true negative rate (TNR)
Specificity refers to the test's ability to correctly reject healthy patients without a condition. Specificity is the result of dividing the true negatives by the sum of the true negatives and false positives (10). Mathematically, this can be expressed as:

Accuracy (ACC)
To calculate the overall accuracy, the number of correctly classified sites should be added up, and then, divided by total number of the reference site (11). Accuracy is the proportion of true results in a population. It measures the accuracy level of a diagnostic test in a condition. The accuracy of a test by definition is its ability to differentiate the patient from healthy cases accurately (12).

Performance evaluation indices
Here are some examples of each index which helps medical researchers for better understanding.
Miss rate (MR) or false negative rate (FNR)
The false negative rate (FNR) is the proportion of positives which yield negative test outcomes with the test, i.e., the conditional probability of a negative test result given that the condition is present (13).

Fall-out or false positive rate (FPR)
In statistics, when performing multiple comparisons, the false positive rate is the probability of falsely rejecting the null hypothesis for a particular test (14).

False discovery rate (FDR)
It is a method of expressing the rate of type I errors in null hypothesis testing when performing multiple comparisons. FDR control procedures are programmed to control FDR. So that the predicted proportion of "discoveries", which are incorrect (15, 16).

False omission rate (FOR)
A negative predictive value refers to that generated by control groups. Meanwhile, the negative probability of the post-test refers to a person's luck. If the individual's pre-test probability is the same as the prevalence in the control group, these two are numerically equal (17).

Informedness or bookmaker informedness (BM)
Informedness is evaluating how regularly the test predicts the result by combining surface measures, and what proportion of the results is correctly predicted. It also introduces markedness as a measure for the estimated probability, which prediction is marked versus chance (18).

Markedness (MK)
Markedness estimates how marked a condition is for the specified predictor, and measures the probability that a condition is marked by the predictor (versus chance). Informedness introduces markedness as a dual measure for this probability; test is marked versus chance (18).

Diagnostic odds ratio (DOR)
DOR is a measure that shows how effective a diagnostic test can be. This is the odds ratio of a positive test. Also about whether the subject has a disease or whether there is a possibility that the test will be positive or not (19).

Positive likelihood ratio (PLR)
The positive likelihood ratio is the probability of a positive test in a patient divided by the probability of a positive test in a healthy person (20).

Negative likelihood ratio (NLR)
It is possible that the test is negative and the person is sick. This is divided by the probability of a negative test for a person who does not have the disease (20).

Prevalence threshold (PT)
This corresponds to the prevalence level below which the positive predictive value of the test is sharply reduced. This is due to the prevalence of the disease and the rate of false positive results can increase (21).

Threat score (TS)
It is the ratio of the area where prediction was accurate, to the area where prediction was not verified (22).

Prevalence
In statistics, prevalence is the proportion of the specific part of population with a special property (23, 24). For example, to calculate the prevalence of malnutrition in a society, the number of people with malnutrition should be divided to the total number of populations. If there are 25 malnourished girls in a population of 100 students in a school, prevalence of malnutrition in that school would be 0.04. This index can be reported as percentage. In that condition, the prevalence will be 4%.

The Fowlkes-mallows (FM)
It is used as a method to determine the similarity between two clusters (the clusters obtained after the clustering algorithm) (25). In other words, this index is a method to indicate the similarity between two clustering (26). A higher value for the Fowlkes-Mallows index indicates greater similarity between clusters and benchmark classifications.

Phi-coefficient or Matthews’s correlation coefficient (MCC)
Phi-coefficient is an index to measure the association between two variables which are binary (27); for example, estimating the association between Rheumatic Heart Disease (RHD) of the blood group types and gender.

F1 score is used as a harmonic mean for recall and precision (28).

Conclusion
To determine the performance of diagnostic tests in medical field, it is necessary to use statistical indicators. In this review study, the most important statistical indicators for evaluating the performance of diagnostic tests and their appropriate use were reviewed. It seems that researchers' familiarity with different disciplines, especially medical sciences, and with performance evaluation indicators, can increase the quality of studies and pave the way for compiling valuable studies.
Acknowledgments
We thank all those who participated in this study and whose valuable comments have strengthened this study.
Conflicts of interest
All authors declare to have no conflict of interest.
Authors’ contributions
The authors all were involved in the whole article but specifically, S. B. and F. M. were involved with Discussion part, F. M., S. B., H. YS., B. F., M. B., and Z. KS. were involved with writing the Results section. F. M. and S. B. was involved with literature review, references, and writing the introduction part of the article.
References
1. Singh JP. Predictive validity performance indicators in violence risk assessment: A methodological primer. Behavioral Sciences & the Law. 2013; 31(1): 8-22.
2. Dziak JJ, Coffman DL, Lanza ST, Li R, Jermiin LS. Sensitivity and specificity of information criteria. Briefings in bioinformatics. 2020; 21(2): 553-65.
3. Vafaie M, Biener M, Mueller M, Schnabel PA, André F, et al. Analytically false or true positive elevations of high sensitivity cardiac troponin: a systematic approach. Heart. 2014; 100(6): 508-14.
4. Garcia JA, Chamorro-Padial J, Rodriguez-Sanchez R, Fdez-Valdivia J. What is the sensitivity and specificity of the peer review process? Accountability in Research. 2022: 1-22.
5. Swift A, Heale R, Twycross A. What are sensitivity and specificity? Evidence-Based Nursing. 2020; 23(1): 2-4.
6. Lee J, Kim KW, Choi SH, Huh J, Park SH. Systematic review and meta-analysis of studies evaluating diagnostic test accuracy: a practical review for clinical researchers-part II. Statistical methods of meta-analysis. Korean journal of radiology. 2015; 16(6): 1188-96.
7. Toft N, Jørgensen E, Højsgaard S. Diagnosing diagnostic tests: evaluating the assumptions underlying the estimation of sensitivity and specificity in the absence of a gold standard. Preventive veterinary medicine. 2005; 68(1): 19-33.
8. Zhu W, Zeng N, Wang N. Sensitivity, specificity, accuracy, associated confidence interval and ROC analysis with practical SAS implementations. NESUG proceedings: health care and life sciences, Baltimore, Maryland. 2010; 19:67.
9. Lalkhen AG, McCluskey A. Clinical tests: sensitivity and specificity. Continuing education in anaesthesia critical care & pain. 2008;8(6):221-3.
10. Chu K. An introduction to sensitivity, specificity, predictive values and likelihood ratios. Emergency Medicine. 1999; 11(3): 175-81.
11. Congalton RG. A review of assessing the accuracy of classifications of remotely sensed data. Remote sensing of environment. 1991; 37(1): 35-46.
12. Gaines P. 14: Accuracy, Precision, Mean and Standard Deviation. Brolin, B. 2004.
13. Saragiotis C, Kitov I, editors. Tuning IMS station processing parameters and detection thresholds to increase detection precision and decrease detection miss rate. EGU General Assembly Conference Abstracts; 2020.
14. Tomar P, Mishra R, Sheoran K. Prediction of quality using ANN based on Teaching‐Learning Optimization in component‐based software systems. Software: Practice and Experience. 2018; 48(4): 896-910.
15. Benjamini Y. Discovering the false discovery rate. Journal of the Royal Statistical Society: series B (statistical methodology). 2010; 72(4): 405-16.
16. Storey JD. False Discovery Rate. International encyclopedia of statistical science. 2011; 1: 504-8.
17. Zafar MB, Valera I, Gomez Rodriguez M, Gummadi KP, editors. Fairness beyond disparate treatment & disparate impact: Learning classification without disparate mistreatment. Proceedings of the 26th international conference on world wide web; 2017.
18. Powers DM. Evaluation: from precision, recall and F-measure to ROC, informedness, markedness and correlation. arXiv preprint arXiv: 201016061. 2020.
19. Glas AS, Lijmer JG, Prins MH, Bonsel GJ, Bossuyt PM. The diagnostic odds ratio: a single indicator of test performance. Journal of clinical epidemiology. 2003; 56(11): 1129-35.
20. Yang Z. Likelihood ratio tests for detecting positive selection and application to primate lysozyme evolution. Molecular biology and evolution. 1998; 15(5): 568-73.
21. Balayla J. Prevalence threshold (ϕ e) and the geometry of screening curves. Plos one. 2020; 15(10): e0240215.
22. Wang C-C. On the calculation and correction of equitable threat score for model quantitative precipitation forecasts for small verification areas: The example of Taiwan. Weather and forecasting. 2014; 29(4): 788-98.
23. Information mh. what is prevalence 2022 [cited 2022 14th January]. Available from: https://www. nimh.nih.gov/health/statistics/what-is-prevalence.
24. Porta M. A dictionary of epidemiology, 5th edition. A call for submissions through an innovative wiki. Journal of Epidemiology and Community Health. 2006; 60(8): 653.
25. Nemec A, Brinkhurst R. The Fowlkes–Mallows statistic and the comparison of two independently determined dendrograms. Canadian Journal of Fisheries and Aquatic Sciences. 1988; 45(6): 971-5.
26. Fowlkes EB, Mallows CL. A Method for Comparing Two Hierarchical Clusterings. Journal of the American Statistical Association. 1983; 78(383): 553-69.
27. Perry NC, Michael WB. The Estimation of a Phi Coefficient for An Entire Criterion Group From a Phi Coefficient Calculated From Use of the Extreme Tails of a Normal Distribution of Criterion Scores. Educational and Psychological Measurement. 1951; 11(4-1): 629-38.
28. Science td. the F1 score 2021 [cited 2022 14th January]. Available from: https://towardsdatascience.com/the-f1-score-bec2bbc38aa6.